Nvidia researchers have developed a new AI image generation technique that could allow highly customized text-to-image models with a fraction of the storage requirements.
According to a paper published on arXiv, the proposed method called “Perfusion” enables adding new visual concepts to an existing model using only 100KB of parameters per concept.
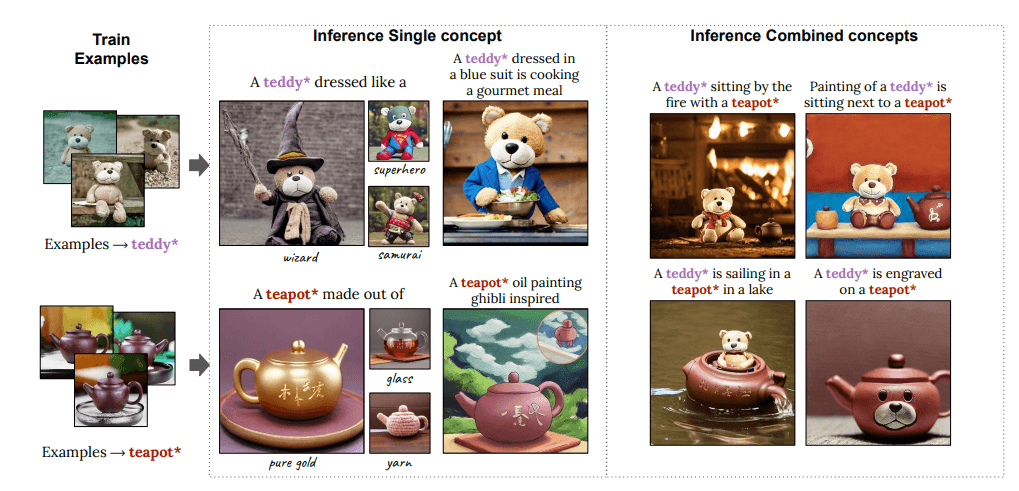
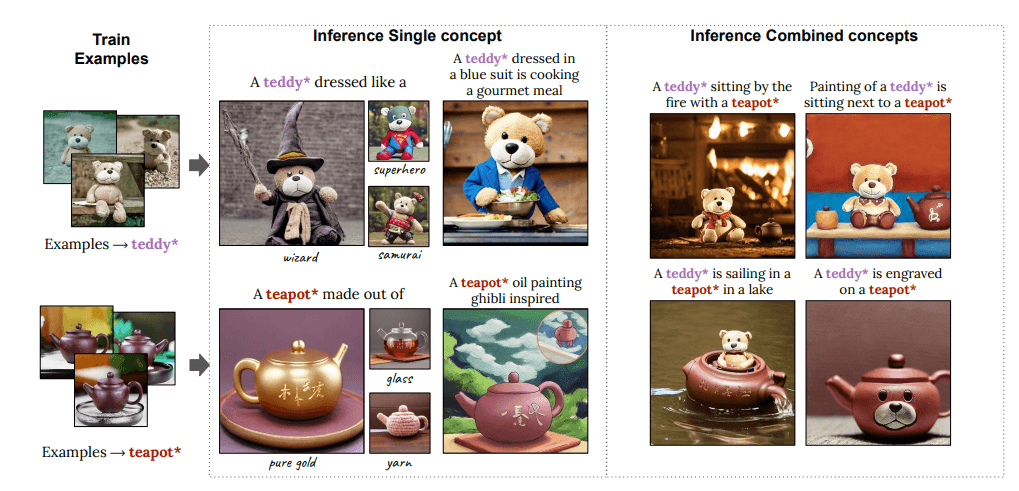
As the paper’s authors describe, Perfusion works by “making small updates to the internal representations of a text-to-image model.”
More specifically, it makes carefully calculated changes to the parts of the model that connect the text descriptions to the generated visual features. Applying minor, parameterized edits to the cross-attention layers allows Perfusion to modify how text inputs get translated into images.
Therefore, Perfusion doesn’t totally retrain a text-to-image model from scratch. Instead, it slightly adjusts the mathematical transformations that turn words into pictures. This allows it to customize the model to produce new visual concepts without needing as much compute power or model retraining.
The Perfusion method needs only 100kb.
Perfusion achieved these results with two to five orders of magnitude fewer parameters than competing techniques.
While other methods may require hundreds of megabytes to gigabytes of storage per concept, Perfusion needs only 100KB – comparable to a small image, text, or WhatsApp message.
This dramatic reduction could make deploying highly customized AI art models more feasible.
According to co-author Gal Chechik,
“Perfusion not only leads to more accurate personalization at a fraction of the model size, but it also enables the use of more complex prompts and the combination of individually-learned concepts at inference time.”
The method allowed creative image generation, like a “teddy bear sailing in a teapot,” using personalized concepts of “teddy bear” and “teapot” learned separately.
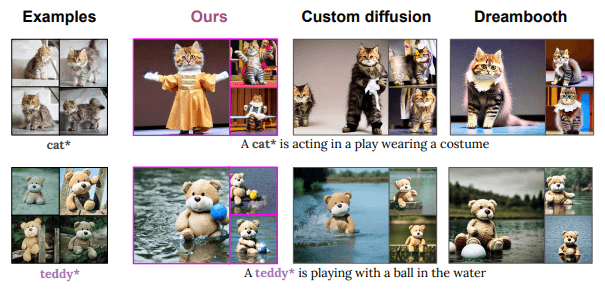
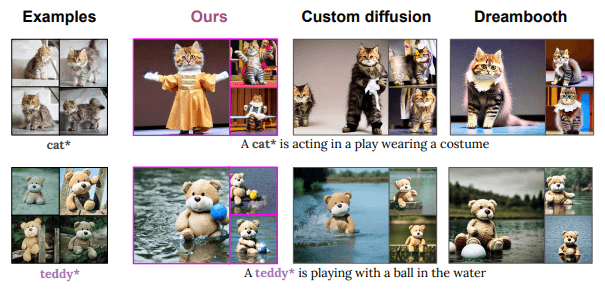
Possibilities of Efficient Personalization
Perfusion’s unique capability to enable the personalization of AI models using just 100KB per concept opens up a myriad of potential applications:
This method paves the way for individuals to easily tailor text-to-image models with new objects, scenes, or styles, eliminating the need for expensive retraining. The efficiency of Perfusion’s 100KB parameter update per concept allows models that are customized with this technique to be implemented on consumer devices, enabling on-device image creation.
One of the most striking aspects of this technique is the potential it offers for sharing and collaboration around AI models. Users could share their personalized concepts as small add-on files, circumventing the need to share cumbersome model checkpoints.
In terms of distribution, models that are tailored to particular organizations could be more easily disseminated or deployed at the edge. As the practice of text-to-image generation continues to become more mainstream, the ability to achieve such significant size reductions without sacrificing functionality will be paramount.
It’s important to note, however, that Perfusion primarily provides model personalization rather than full generative capability itself.
Limitations and Release
While promising, the technique does have some limitations. The authors note that critical choices during training can sometimes over-generalize a concept. More research is still needed to seamlessly combine multiple personalized ideas within a single image.
The authors note that code for Perfusion will be made available on their project page, indicating an intention to release the method publicly in the future, likely pending peer review and an official research publication. However, specifics on public availability remain unclear since the work is currently only published on arXiv. On this platform, researchers can upload papers before formal peer review and publication in journals/conferences.
While Perfusion’s code is not yet accessible, the authors’ stated plan implies that this efficient, personalized AI system could find its way into the hands of developers, industries, and creators in due course.
As AI art platforms like MidJourney, DALL-E 2, and Stable Diffusion gain steam, techniques that allow greater user control could prove critical for real-world deployment. With clever efficiency improvements like Perfusion, Nvidia appears determined to retain its edge in a rapidly evolving landscape.
Comments (No)